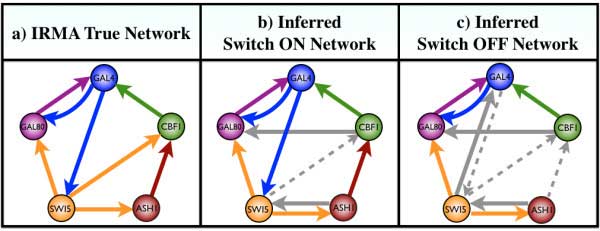
A new paper by Dr. M. Paola Vera-Licona and Dr. Reinhard Laubenbacher entitled “An algebra-based method for inferring gene regulatory networks” published in BMC Systems Biology has been noted as an Editor’s pick. It’s also ranked as a highly accessed article. The paper, found in BMC Systems Biology 2014, 8:37, describes a new gene regulatory network inference method based on polynomial dynamical systems incorporates time-resolved gene expression data and existing causal information and into its model inference and uses and evolutionary algorithm for parameter estimation. Congratulations Dr. Vera-Licona and Laubenbacher! Check out the paper at www.biomedcentral.com/1752-0509/8/37.
Paola Vera-Licona, Abdul Jarrah, Luis David Garcia-Puente, John McGee, Reinhard Laubenbacher. An algebra-based method for inferring gene regulatory networks. BMC Syst Biol. 2014; 8: 37. Published online 2014 March 26. doi: 10.1186/1752-0509-8-37.
Download a full text PDF version of An algebra-based method for inferring gene regulatory networks.